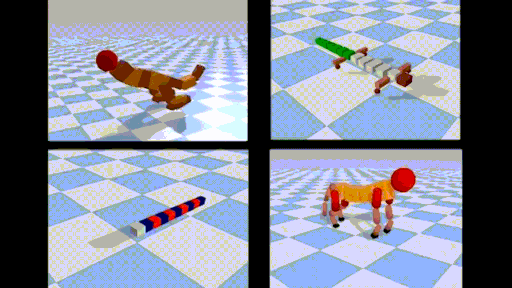
Low Dimensional Motor Skill Learning Using Coactivation
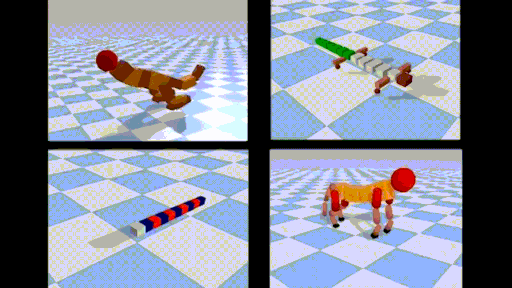
Locomotion in humans and animals is highly coordinated, with many joints moving together. Learning similar coordinated locomotion in articulated virtual characters, in the absence of reference motion data, is a challenging task due to the high number of degrees of freedom and the redundancy that comes with it. In this paper, we present a method for learning locomotion for virtual characters in a low dimensional latent space which defines how different joints move together. We introduce a technique called motor babble, wherein a character interacts with its environment by actuating its joints through uncoordinated, low-level (motor) excitations, resulting in a corpus of motion data from which a manifold latent space is extracted. Dimensions of the extracted manifold define a wide variety of synergies pertaining to the character and, through reinforcement learning, we train the character to learn locomotion in the latent space by selecting a small set of appropriate latent dimensions, along with learning the corresponding policy.
Highlights Video
Publications
A. Ranganath A. Biswas, I. Karamouzas, and V Zordan, “Motor Babble: Morphology Driven Coordinated Control of Articulated Characters”, in Motion, Interaction and Games, 2021, Lausanne, Switzerland, Nov 2021. [PDF]